Using deep learning to predict clinical outcomes in heart failure
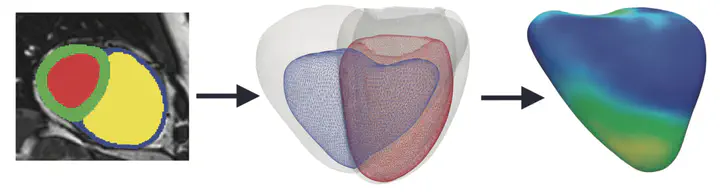
This project will investigate human heart failure, which is a complex clinical syndrome associated with differing adaptations of ventricular contractility and geometry as a consequence of altered loading conditions, intrinsic myocardial disease and ischaemia. Although prognosis depends on eventual ventricular decompensation, conventional measures of cardiac function are often poor predictors of outcome in individual patients. Three-dimensional cardiac phenotyping coupled with advanced segmentation and deep learning (DL) algorithms offers a powerful new approach for risk stratification which can be trained on large cohorts of patients with known outcomes. We will determine if DL approaches allow high-precision prediction of individual outcomes by exploiting both complex cardiac phenotypes and conventional risk factors. We will apply this to patient cohorts with dilated cardiomyopathy and pulmonary arterial hypertension. To determine the true predictive performance in unseen patients outcome prediction will be externally validated on an independent cohort and compared to contemporary risk prediction models. The expected result is a validated technique of autonomous image interpretation to predict outcomes and guide management. This work will lay the foundation for introducing DL to decision-making and risk prediction in cardiology by exploiting massive multimodal datasets for classifier training.